The Rise of Vertical AI and the Value of Efficient Domain Models
The imperative for companies to adopt Artificial Intelligence (AI) solutions is intensifying. However, the path to effective implementation and productionalizing models presents challenges, especially depending on industry. While generalizable and commercial off-the-shelf AI models offer a broad foundation, their lack of specialization (e.g., specific financial or healthcare regulatory knowledge) and frequent hallucinations often necessitates extensive fine-tuning and substantial datasets, leading to protracted development cycles and inflated costs. This post explores the growing development of “Vertical AI”, focusing on the utilization of efficient domain models as a key driver of AI adoption and ROI.
Vertical AI, characterized by its focus on specific industries or business functions, presents a compelling alternative to general approaches. By leveraging pre-trained models imbued with domain-specific knowledge, companies can unlock a range of benefits using their proprietary data and knowledge, including streamlined development, reduced costs, and improved accuracy and fewer hallucinations. This targeted approach allows for the development of bespoke AI solutions that address unique industry challenges and drive competitive advantage.
Efficiency through Specialization: The Role of Domain Models
Domain models are purpose-built AI models trained on data relevant to a particular industry or function. Unlike generic models, which require extensive customization and fine-tuning to achieve optimal performance, domain models arrive pre-equipped with a wealth of specialized knowledge and can be fine-tuned even more with proprietary data that is owned end-to-end by the domain experts. This inherent efficiency translates to several key advantages:
- Accelerated Development Cycles: By leveraging domain models as a foundation, development teams can significantly reduce the time and resources required for training and fine-tuning models. This accelerated time-to-value allows for rapid deployment and faster realization of ROI.
- Cost Optimization: Building AI solutions from scratch is a resource-intensive endeavor. Domain models offer a more cost-effective alternative by providing a pre-existing framework upon which to build. This reduces initial investment and allows for the allocation of resources to other critical areas.
- Enhanced Accuracy and Relevance: Generic AI models, trained on vast and generalized datasets, often struggle to achieve high accuracy in specialized domains. Domain models, conversely, are trained on curated data specific to the industry or function, leading to improved accuracy, more relevant insights, and superior performance on domain-specific tasks. They can be further fine-tuned with proprietary data to be even more accurate and performant.
Evidentiary Support and Practical Applications
The efficacy of domain-specific models is supported by a growing body of research. For instance, the "LoRA: Low-Rank Adaptation of Large Language Models" (Hu et al., 2021) paper demonstrates how parameter-efficient fine-tuning techniques can be applied to large language models to achieve strong performance on specific tasks with minimal computational overhead. Fine-tuning a 7B parameter model can now be done in a single GPU rather than a cluster. There are additional papers that show new techniques (e.g., DeepSeek) for smaller, intentionally tuned models can perform just as well as larger models or better at the given task. This approach exemplifies the trend towards specialized models that deliver targeted results, as now many such models can be chained together for a task-specific approach.
Furthermore, real-world applications of vertical AI are emerging across diverse sectors. In Healthcare, domain specific LLMs are being fine-tuned or as the basis of RAG models to improve administrative and clinical decision making tasks, and serving as a source of intelligence for healthcare data generally for different stakeholders (e.g., translating a pathology report into easier-to-understand verbiage for patients). For the legal industry, custom LLMs are being built to handle legal and consulting tasks such as research or specific question-answering across documents. Some approaches even build context-aware models per document. Last, in Finance, co-pilots for financial advisors and automation in underwriting, tax, and auditing are being developed with specific regulatory and tax code knowledge.
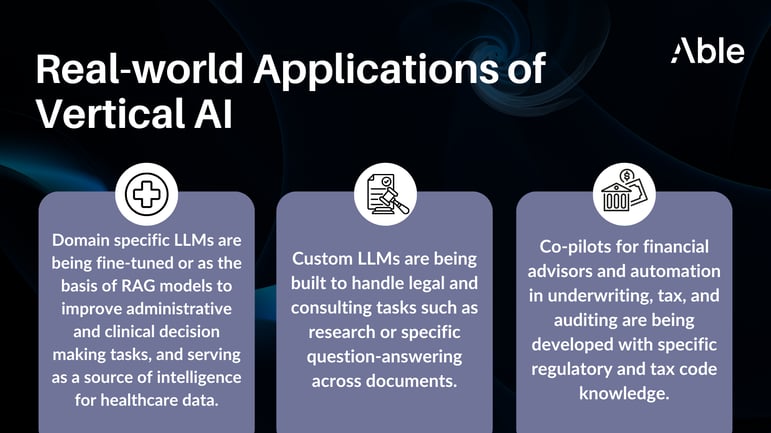
Strategic Considerations for Mid-Market CTOs
When evaluating vertical AI solutions, which may have more complex implementations relative to using off-the-shelf general models, CTOs should consider the following approaches in addition to standard strategic business criteria:
Proof of Concept (POC): Consider conducting a pilot project to test the solution in a real-world setting with practical workflows that integrate into business priorities before committing to a full-scale implementation. Clearly define success criteria.
Cost-Benefit Analysis: Thoroughly analyze the costs and benefits of the AI solution, considering both short-term and long-term implications. Consider the changes in research, which is trending toward even more efficient and reduced constraints on compute and hardware for such tasks.
Internal Expertise: Vertical models may require additional skills to implement or maintain. Assess your internal team's capabilities and identify any skills gaps that need to be addressed, as well as any change management efforts.
Conclusion
The rise of vertical AI marks a significant evolution in the AI landscape. By leveraging efficient domain models, mid-market companies can overcome the hallucinations and limitations of generic AI solutions and unlock more specialized models fit for industry tasks. As the demand for specialized AI solutions grows, CTOs who embrace this trend will be well-positioned to drive innovation, optimize operations, and gain a decisive competitive edge in an era where startups and innovators are using domain models to disrupt industries.
Michelle Yi, Head of Applied AI
Michelle brings over 15 years of experience in machine learning, cloud computing, and AI innovation. A passionate advocate for diversity in STEM and community engagement, Michelle specializes in leveraging AI to tackle complex challenges and drive meaningful impact across industries.